Statistical Methods for Recommender Systems: A Comprehensive Exploration

4.5 out of 5
Language | : | English |
File size | : | 12992 KB |
Text-to-Speech | : | Enabled |
Screen Reader | : | Supported |
Enhanced typesetting | : | Enabled |
Print length | : | 297 pages |
Recommender systems are ubiquitous in today's digital world, powering personalized recommendations for products, movies, music, news articles, and more. They are designed to help users navigate the vast ocean of information available online and discover items that they are likely to enjoy.
Statistical methods play a vital role in the development and improvement of recommender systems. These methods are used for data preprocessing, similarity measures, prediction algorithms, and evaluation metrics. By leveraging statistical techniques, recommender systems can be made more accurate, efficient, and personalized.
In this article, we will provide a comprehensive exploration of statistical methods used in recommender systems. We will cover the following topics:
* Data preprocessing * Similarity measures * Prediction algorithms * Evaluation metrics
Data Preprocessing
The first step in building a recommender system is to preprocess the data. This involves cleaning the data, removing duplicate and erroneous data points, and transforming the data into a format that is suitable for recommender algorithms.
Statistical methods can be used for data preprocessing in a variety of ways. For example, descriptive statistics can be used to identify missing values and outliers. Statistical tests can be used to determine whether two variables are correlated. And data transformation techniques can be used to improve the accuracy of recommender algorithms.
Similarity Measures
Similarity measures are used to quantify the similarity between two users or items. This information is then used by recommender algorithms to make predictions.
There are many different statistical methods that can be used to calculate similarity measures. Some of the most common methods include:
* Pearson correlation coefficient * Spearman rank correlation coefficient * Jaccard similarity coefficient * Cosine similarity * Euclidean distance
The choice of similarity measure depends on the type of data being used and the specific recommender algorithm that is being employed.
Prediction Algorithms
Prediction algorithms are used to predict the rating that a user would give to an item. This information is then used to generate personalized recommendations.
There are many different statistical methods that can be used for prediction in recommender systems. Some of the most common methods include:
* Collaborative filtering * Content-based filtering * Hybrid recommender systems
Collaborative filtering algorithms use data about user-item interactions to make predictions. Content-based filtering algorithms use data about the properties of items to make predictions. And hybrid recommender systems combine elements of both collaborative filtering and content-based filtering.
Evaluation Metrics
Evaluation metrics are used to assess the performance of recommender systems. These metrics measure the accuracy, efficiency, and personalization of recommender algorithms.
There are many different evaluation metrics that can be used for recommender systems. Some of the most common metrics include:
* Mean absolute error (MAE) * Root mean squared error (RMSE) * Precision * Recall * F1 score
The choice of evaluation metric depends on the specific goals of the recommender system.
Statistical methods are essential for the development and improvement of recommender systems. These methods are used for data preprocessing, similarity measures, prediction algorithms, and evaluation metrics. By leveraging statistical techniques, recommender systems can be made more accurate, efficient, and personalized.
As the field of recommender systems continues to evolve, new statistical methods will be developed to address the challenges of big data, personalization, and real-time recommendations. By staying up-to-date on the latest statistical methods, you can ensure that your recommender systems are delivering the best possible user experience.
4.5 out of 5
Language | : | English |
File size | : | 12992 KB |
Text-to-Speech | : | Enabled |
Screen Reader | : | Supported |
Enhanced typesetting | : | Enabled |
Print length | : | 297 pages |
Do you want to contribute by writing guest posts on this blog?
Please contact us and send us a resume of previous articles that you have written.
Novel
Page
Chapter
Text
Genre
Library
Paperback
E-book
Newspaper
Paragraph
Sentence
Bookmark
Shelf
Bibliography
Preface
Synopsis
Footnote
Manuscript
Scroll
Codex
Tome
Narrative
Memoir
Dictionary
Narrator
Archives
Periodicals
Study
Research
Lending
Journals
Rare Books
Literacy
Study Group
Thesis
Storytelling
Awards
Book Club
Theory
Textbooks
Collette Cameron
Rod Decker
Adam Dodek
Random House
Rilla Askew
Jee Utrecht
Adam Gamble
Li Chen
G Cristina Mora
Adam Ferrier
Shelly Down
Max Hennessy
Robert G Natelson
Fabrice Mocellin
Kevin Emerson
Cheryl R Cowtan
Elliot Miles Emery
Jim Marshall
Odin Redbeard
Alvah Hovey
Light bulbAdvertise smarter! Our strategic ad space ensures maximum exposure. Reserve your spot today!
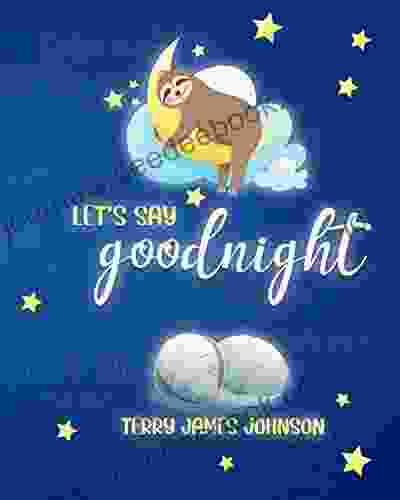

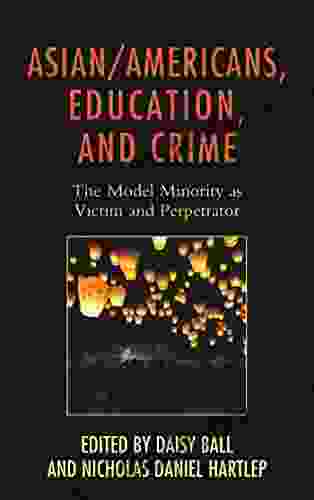

- Dylan MitchellFollow ·12.5k
- Hugh BellFollow ·7.1k
- Benjamin StoneFollow ·15.2k
- Ralph TurnerFollow ·6k
- Ervin BellFollow ·5.8k
- Simon MitchellFollow ·8.1k
- Troy SimmonsFollow ·6k
- Kazuo IshiguroFollow ·13.3k
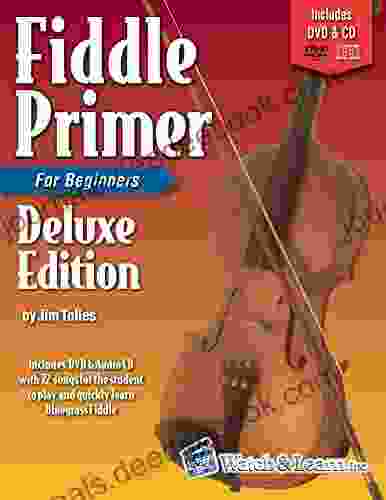

Fiddle Primer for Beginners Deluxe Edition: Your...
Embark on an...
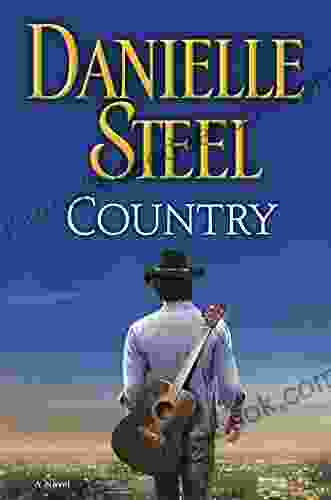

An Enchanting Journey into the Alluring World of Danielle...
Danielle Steel is an American...
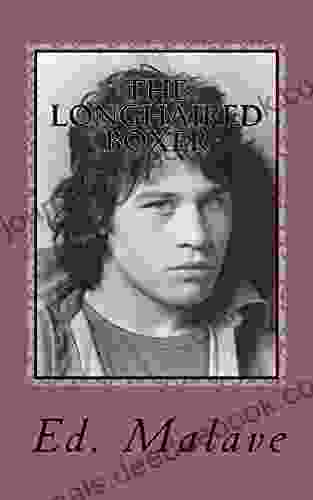

The Longhaired Boxer: Ed Malave and His Legacy in the...
Ed Malave, known...
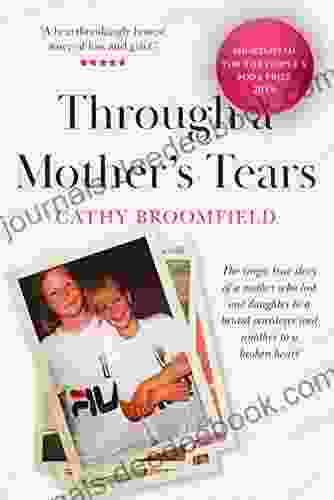

The Tragic True Story Of A Mother Who Lost One Daughter...
No parent should...
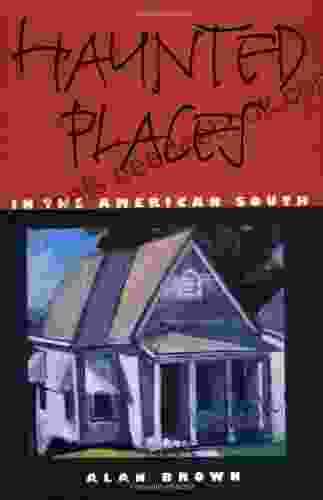

Haunted Places In The American South: An Exploration of...
As the sun dips...
4.5 out of 5
Language | : | English |
File size | : | 12992 KB |
Text-to-Speech | : | Enabled |
Screen Reader | : | Supported |
Enhanced typesetting | : | Enabled |
Print length | : | 297 pages |