PyTorch Reinforcement Learning Cookbook: A Comprehensive Guide to Building Intelligent Agents

4.7 out of 5
Language | : | English |
File size | : | 9967 KB |
Text-to-Speech | : | Enabled |
Screen Reader | : | Supported |
Enhanced typesetting | : | Enabled |
Print length | : | 527 pages |
Reinforcement learning (RL) is a type of machine learning that allows agents to learn how to behave in an environment by interacting with it and receiving rewards or punishments for their actions. RL has been used to create agents that can play games, control robots, and even trade stocks.
PyTorch is a popular deep learning library that is well-suited for RL. It provides a number of tools that make it easy to build and train RL models.
This cookbook provides a comprehensive guide to building RL models with PyTorch. It covers a wide range of topics, including:
- Model-based reinforcement learning
- Model-free reinforcement learning
- Hybrid reinforcement learning
- Advanced RL techniques
This cookbook is written for both beginners and experienced RL practitioners. Beginners will find the step-by-step instructions and clear explanations helpful. Experienced RL practitioners will find the advanced techniques and case studies valuable.
Model-based reinforcement learning
Model-based reinforcement learning is a type of RL that uses a model of the environment to make decisions. This model can be used to predict the consequences of different actions, which allows the agent to make more informed decisions.
PyTorch provides a number of tools that make it easy to build and train model-based RL models. These tools include:
- The
torch.nn
module provides a number of neural network building blocks that can be used to build models of the environment. - The
torch.optim
module provides a number of optimization algorithms that can be used to train models. - The
torch.distributions
module provides a number of probability distributions that can be used to model the uncertainty in the environment.
The following code shows how to build a simple model-based RL model with PyTorch:
python import torch import torch.nn as nn import torch.optim as optim import torch.distributions as distributions
# Define the environment model class EnvironmentModel(nn.Module): def __init__(self): super(EnvironmentModel, self).__init__() self.fc1 = nn.Linear(4, 64) self.fc2 = nn.Linear(64, 64) self.fc3 = nn.Linear(64, 1)
def forward(self, state): x = F.relu(self.fc1(state)) x = F.relu(self.fc2(x)) x = self.fc3(x) return x
# Define the RL agent class RLAgent(nn.Module): def __init__(self): super(RLAgent, self).__init__() self.fc1 = nn.Linear(4, 64) self.fc2 = nn.Linear(64, 64) self.fc3 = nn.Linear(64, 1)
def forward(self, state): x = F.relu(self.fc1(state)) x = F.relu(self.fc2(x)) x = self.fc3(x) return x
# Define the training loop def train(env, agent, model, optimizer, num_episodes): for episode in range(num_episodes): # Reset the environment state = env.reset()
# Loop until the episode is finished while True: # Get the agent's action action = agent(state)
# Take the action and observe the reward and next state next_state, reward, done, _ = env.step(action)
# Update the model loss = F.mse_loss(model(state),reward) optimizer.zero_grad() loss.backward() optimizer.step()
# Update the state state = next_state
# Check if the episode is finished if done: break
# Create the environment env = gym.make('CartPole-v0')
# Create the agent agent = RLAgent()
# Create the model model = EnvironmentModel()
# Create the optimizer optimizer = optim.Adam(model.parameters())
# Train the model train(env, agent, model, optimizer, 1000)
Model-free reinforcement learning
Model-free reinforcement learning is a type of RL that does not use a model of the environment. Instead, it learns directly from experience.
PyTorch provides a number of tools that make it easy to build and train model-free RL models. These tools include:
- The
torch.nn
module provides a number of neural network building blocks that can be used to build RL models. - The
torch.optim
module provides a number of optimization algorithms that can be used to train models. - The
torch.distributions
module provides a number of probability distributions that can be used to model the uncertainty in the environment.
The following code shows how to build a simple model-free RL model with PyTorch:
python import torch import torch.nn as nn import torch.optim as optim import torch.distributions as distributions
# Define the RL agent class RLAgent(nn.Module): def __init__(self): super(RLAgent, self).__init__() self.fc1 = nn.Linear(4, 64) self.fc2 = nn.Linear(64, 64) self.fc3 = nn.Linear(64, 1)
def forward(self, state): x = F.relu(self.fc1(state)) x = F.relu(self.fc2(x)) x = self.fc3(x) return x
# Define the training loop def train(env, agent, optimizer, num_episodes): for episode in range(num_episodes): # Reset the environment state = env.reset()
# Loop until the episode is finished while True: # Get the agent's action action = agent(state)
# Take the action and observe the reward and next state
4.7 out of 5
Language | : | English |
File size | : | 9967 KB |
Text-to-Speech | : | Enabled |
Screen Reader | : | Supported |
Enhanced typesetting | : | Enabled |
Print length | : | 527 pages |
Do you want to contribute by writing guest posts on this blog?
Please contact us and send us a resume of previous articles that you have written.
Novel
Chapter
Text
Paperback
E-book
Magazine
Paragraph
Sentence
Bookmark
Shelf
Bibliography
Foreword
Footnote
Manuscript
Scroll
Codex
Bestseller
Classics
Narrative
Reference
Encyclopedia
Dictionary
Thesaurus
Character
Resolution
Librarian
Catalog
Card Catalog
Archives
Periodicals
Scholarly
Reserve
Academic
Reading Room
Rare Books
Study Group
Thesis
Dissertation
Storytelling
Reading List
Karl Kemp
Pierre Gilles Lemarie Rieusset
Adam Ferrier
Cristiano Nogueira
Paul Masterdon
Anthony Barnett
Marion Clarion
Lane Lasater
Danielle Ogier
Tom Hope
Esteban Navarro
Michael I Harrison
Petrina Engelke
Gina Latimerlo
Ernest Ialongo
Maynard Webb
Elliot Miles Emery
Melanie Linn Gutowski
Laure Eve
Aleta Williams
Light bulbAdvertise smarter! Our strategic ad space ensures maximum exposure. Reserve your spot today!
- Joe SimmonsFollow ·16.6k
- Jayden CoxFollow ·17.3k
- Gage HayesFollow ·11.5k
- Jeffrey CoxFollow ·9.5k
- Lord ByronFollow ·8k
- Pat MitchellFollow ·14.5k
- Nick TurnerFollow ·11.9k
- Duane KellyFollow ·9.6k
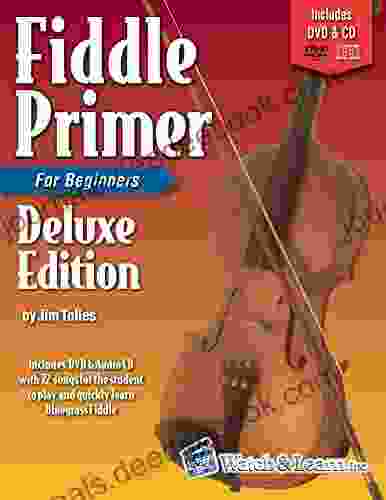

Fiddle Primer for Beginners Deluxe Edition: Your...
Embark on an...
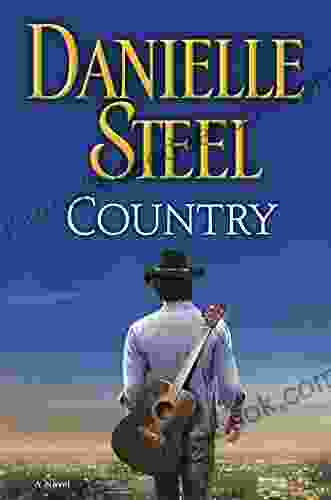

An Enchanting Journey into the Alluring World of Danielle...
Danielle Steel is an American...
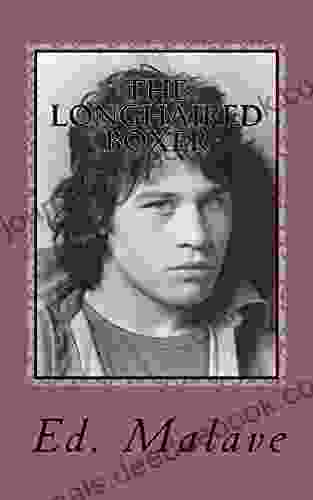

The Longhaired Boxer: Ed Malave and His Legacy in the...
Ed Malave, known...
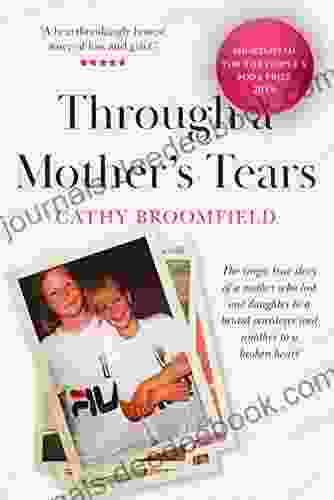

The Tragic True Story Of A Mother Who Lost One Daughter...
No parent should...
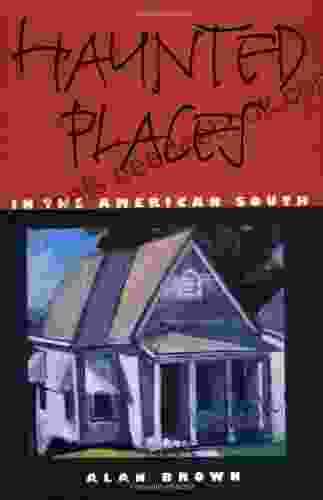

Haunted Places In The American South: An Exploration of...
As the sun dips...
4.7 out of 5
Language | : | English |
File size | : | 9967 KB |
Text-to-Speech | : | Enabled |
Screen Reader | : | Supported |
Enhanced typesetting | : | Enabled |
Print length | : | 527 pages |